Due to the war in Ukraine and the global pandemic, agriculture is going through a severe turmoil. The price of fuel is increasing and the price of fertiliser and pesticide has even tripled in some cases. On the other hand, the global population is expected to reach 8 bn by 15 November. This means that farmers are under huge pressure to produce more with less. Previous agricultural revolutions in history were driven by the advances in mechanical and chemical industry, but today, it is information technologies that have the power to change the way we are producing food.
The first step in any kind of improvement of agricultural production is always monitoring. It reveals the weak spots of the production and helps us steer our efforts where they are needed most. Traditionally, monitoring is done by visual inspection of the farmer, but this is very subjective, slow and unreliable. For this reason, satellites, drones and sensors are used more and more. The FlexiGroBots project is mainly based on UAV monitoring as these devices can give us high-resolution data in different phenological phases of crop in multiple spectral channels.
Human eyes have three colour sensors - red, green and blue, and any colour that we see is a distinct mixture of these. It is only when we add infrared, red edge and other specific colours (or wavelengths) that we can see the full picture of what is going on in the field. However, in order to process this vast amount of information from images, we need AI. For this reason we are developing complex deep learning algorithms that can assess the crop growth, detect nutrient/water deficiencies, and detect different areas inside the field. These areas can be the patches of weed, zones affected by plant diseases or just the regular plants.
These deep learning algorithms are usually quite complex and in order to be trained for a particular use-case, they not only need to be fed with appropriate data, but also need to be tweaked and fine-tuned in terms of their architecture. This is a very tedious task even for a data scientist, as she needs to explore architectures with different numbers of layers and neurons, and types of network elements. For this reason, we are designing an AI toolbox with integrated AutoML functionality. What this means is that the user can just enter the data, press the button and immediately get an optimal model for a particular use-case.
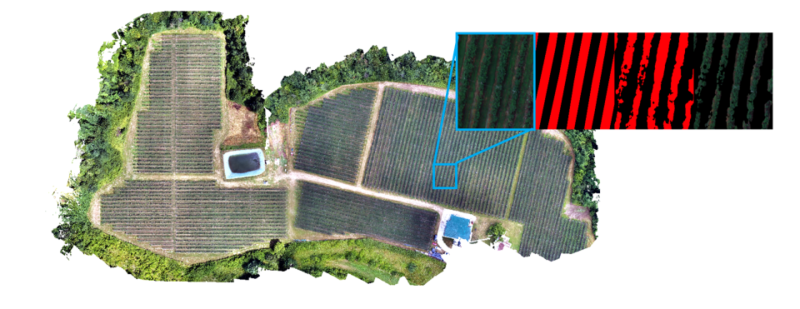
Under the hood, the AutoML functionality explores a number of different state-of-the-art architectures and their variants and finds the one, most suitable for the problem. These architectures come as brand new deep learning models or come as models “prefabricated” for general purpose image processing, which are tweaked to fit the given scenario. This approach has already given some very interesting results. The deep learning approach was attempted in Pilot 3, for detection of blueberry rows and the inter-row spacing. Row detection allows us to analyse the plants and not the grass between them, while the detection of passages has allowed us to define routes along which the ground robot should move to perform the soil sampling and weed spraying tasks.
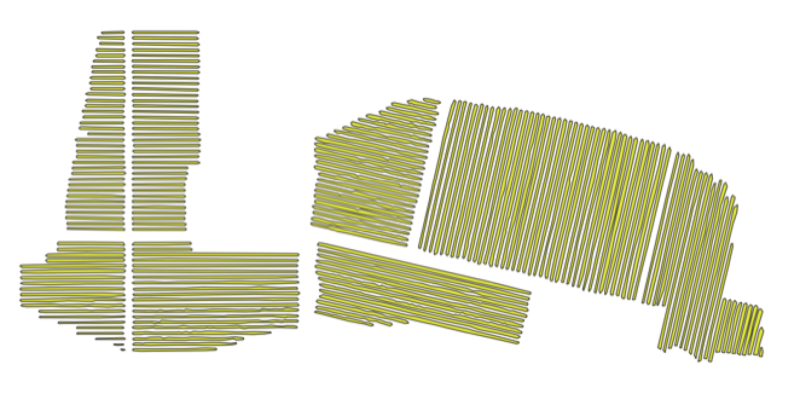
The next step in the AI Toolbox development is to apply the same approach in other pilots, for the analysis of rapeseed and vineyard images, as well as images and videos taken by cameras onboard the robotic vehicles. This will demonstrate the effectiveness and scalability of the system, making it a crucial component of the complex system of flexible robots in agriculture.