Crop diseases can cause significant loss of yield and quality of the final product. Therefore, detecting them at an early stage reduces the likelihood of spread around the field. Moreover, the application of pesticide only on infected plants lowers the amount of chemical employed, which is beneficial for the environment.
Pilot 1 from FlexiGroBots is working on developing in a system for early detection of a fungal disease called Botrytis bunch rot or gray mold, caused by Botrytis cinerea. This disease not only affects grapevines, but also other crops such as strawberries and tomatoes.
Wageningen University (WU) and the Spanish National Research Council (CSIC) are the partners performing this detection task. WU is responsible for the aerial fleet of drones or Unmanned Aerial Vehicles (UAVs) and CSIC is responsible for the fleet of ground robots or Unmanned Ground Vehicles (UGVs).
Aerial and ground robots will work collaboratively to accurately detect this pest.
Wageningen University acquires aerial images taken with the UAV at different phenological stages of the vineyard, starting before veraison and ending at harvesting. The main objective is to identify where Botrytis will develop. The algorithm under development intakes several variables such as the Digital Terrain Model (DTM), the Digital Surface Model (DSM), the Leaf Area Index (LAI), and some vegetation indices (VIs), and outputs a risk map or heatmap with the areas which have the highest likelihood of being affected by Botrytis. This heatmap is then sent to the UGVs.
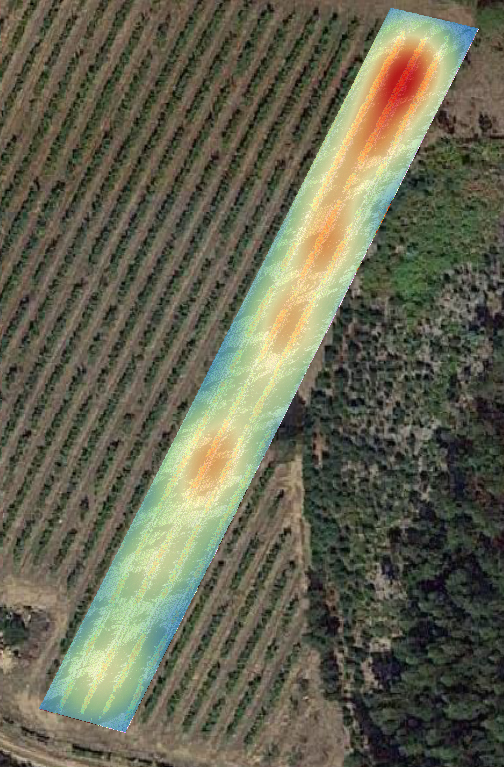
The CSIC is developing a system that takes the heat map obtained from aerial robots and generates scouting routes for the ground robots so that they will go around the vineyard scouting the whole crop but paying more attention to the areas where there is more risk of finding Botrytis. The detection of Botrytis from ground robots' images is carried out using a convolutional neural network (CNN) that is currently being trained using machine learning techniques, specifically Deep Learning techniques. In the first week of August, images of grapes affected and not affected by Botrytis will be collected in order to continue training the CNN with new datasets that collects images from an early stage of the disease. In the future, the aim is to connect the detection network with the treatment system in order to achieve an early detection and precision treatment of the disease. The detection and treatment systems will be on board ground robots.
The ground robots are based on commercial electric vehicles with a range of 80 km, which have been robotized so that they can perform an agricultural task, in this case scouting, autonomously (see figure 2).
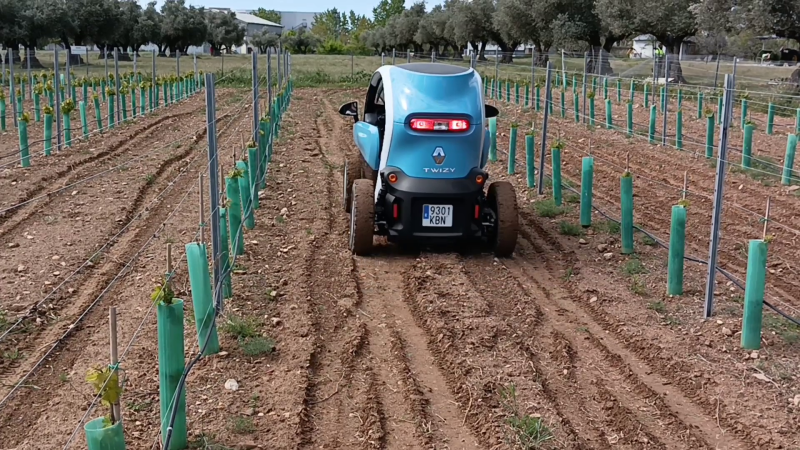
Wageningen University Team (WU)
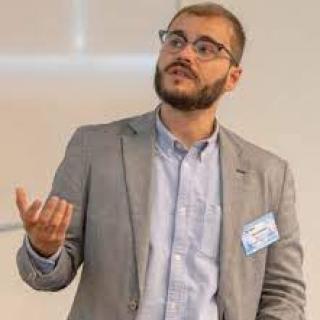
Dr. João Valente
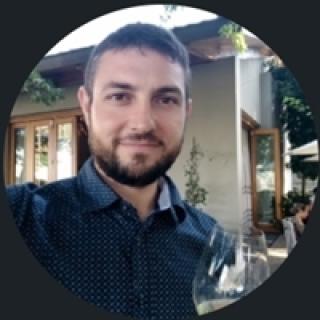
Dr. Sergio Vélez Martín
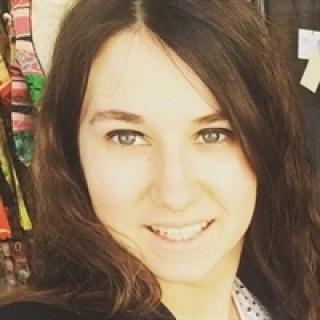
MSc. Mar Ariza-Sentís
CSIC (Spanish National Research Council) Team
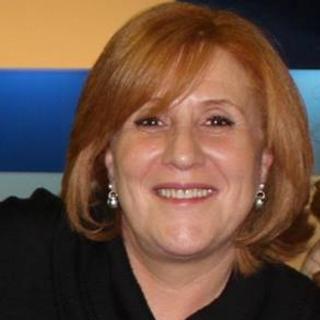
Dr. Angela Ribeiro
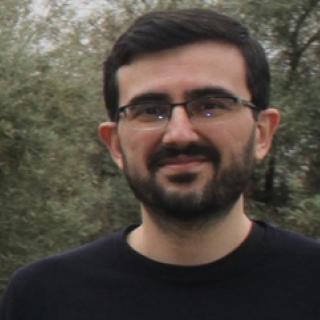
Dr. José Maria Bengochea-Guevara
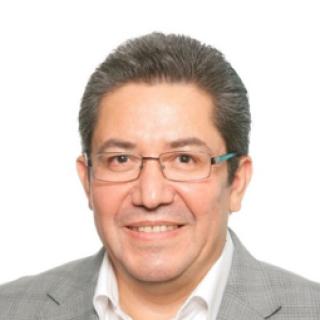
Dr. Hector Montes
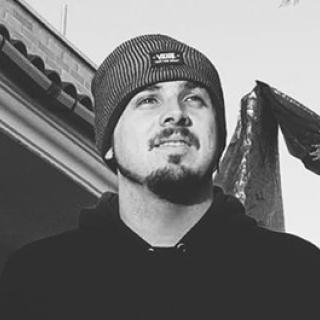